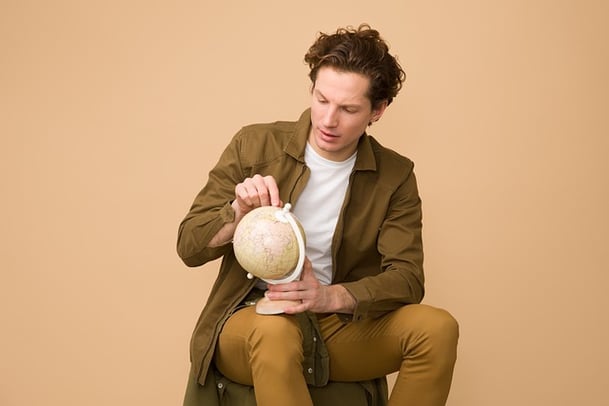
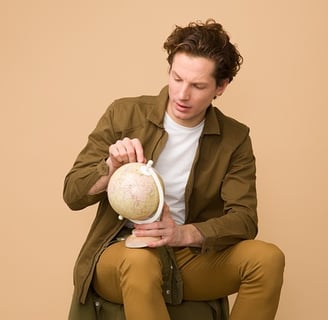
Researcher | Lifelong Learning AI | Neuromorphic Computing
ASHLEY GREEN
I specialize in leveraging GPT-4 fine-tuning to develop lifelong learning AI systems that mitigate catastrophic forgetting through neuromorphic hardware-inspired approaches and dynamic memory architectures. My research focuses on enabling AI to continuously acquire new knowledge while retaining and optimizing prior learning, fostering the development of efficient and robust long-term intelligent systems.
By integrating deep learning, brain-inspired computing, and reinforcement learning, I aim to advance AI applications in personalized education platforms and adaptive cybersecurity. I am passionate about the potential of federated learning, generative memory models, and self-supervised learning in enhancing AI adaptability and sustainable learning, striving to contribute both theoretical and practical advancements to the future of intelligent systems.
Integration of Neuromorphic Principles
Provide a blueprint for incorporating biological plasticity and dynamic memory architectures into AI systems, improving their ability to handle incremental tasks
Applications in Society
Enable personalized education platforms that adapt to individual learning needs and adaptive cybersecurity systems that evolve to counter emerging threats, addressing critical societal challenges.
Advancement of Lifelong Learning AI
Demonstrate how GPT-4 fine-tuning can enhance the development of AI systems capable of continuous learning without catastrophic forgetting, setting a new standard for adaptability.
Contribute to the development of AI systems that are not only technically advanced but also ethically sound and aligned with societal values.